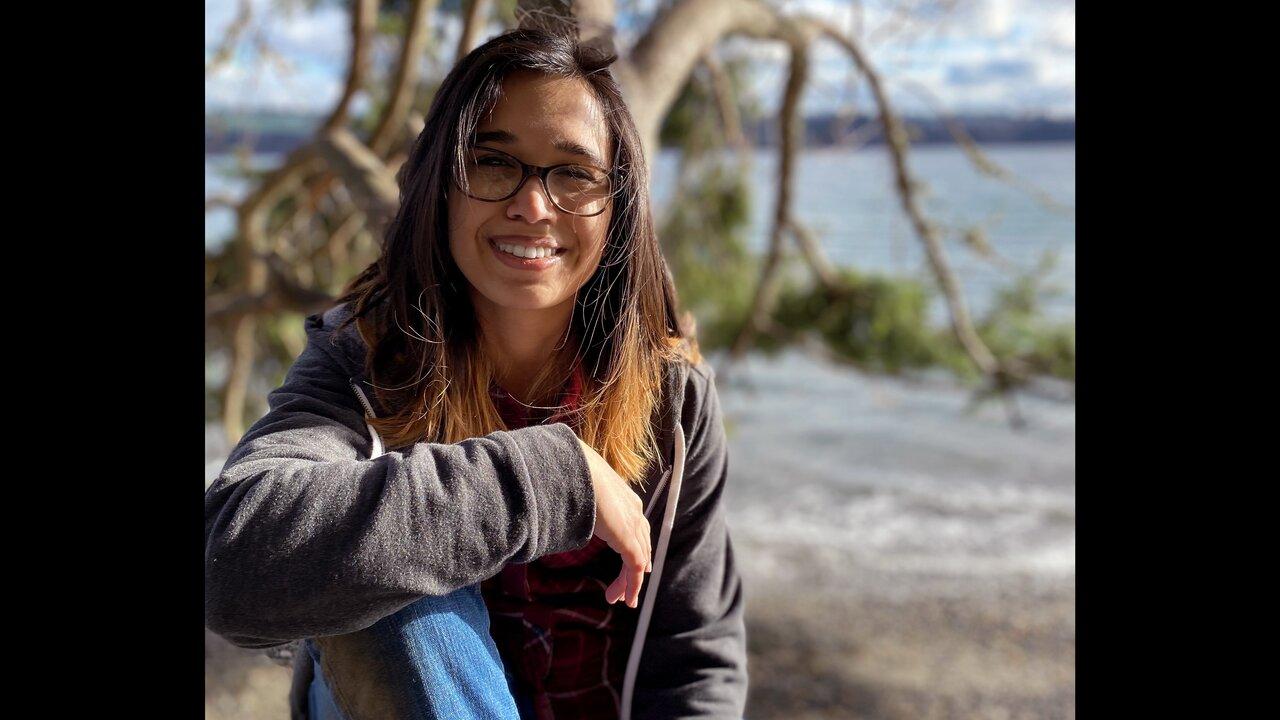
Ph.D. Student Meghna Srivastava Receives NSF Graduate Research Fellowship
Second-year materials science and engineering (MSE) Ph.D. student Meghna Srivastava has received a National Science Foundation (NSF) Graduate Research Fellowship for her research on perovskites, a promising class of materials for next-generation energy devices.
The NSF Graduate Research Fellowship Program (GRFP) is the oldest and one of the most prestigious fellowships of its kind. It recognizes and supports outstanding graduate students in STEM who have the potential to become knowledge experts and significantly contribute to research, teaching and innovation.
“It is a really nice personal honor to be selected for this, but my advisor and my lab helped a lot with this, so I’m happy for them too, and I feel that we all get something out of it,” said Srivastava. “It’s also great to see that the NSF believes my work has the potential to contribute to the field and the world.”
Srivastava works in MSE associate professor Marina Leite’s lab, studying ways to use machine learning to study perovskites. Perovskites convert solar energy very efficiently, but are susceptible to degradation and are highly sensitive to environmental factors like heat, light, humidity and electrical bias. This makes developing a stable and durable perovskite solar cell an important challenge for making solar energy accessible. However, finding the right materials is difficult.
“The compositional space for perovskites is enormous,” she said. “There are three sites in the [perovskite] crystal structure, and each site can have different combinations of ions, so there is just an insane number of possible combinations. It’s a lot of trial and error.”
A New Tool for Studying Materials
One possible solution is machine learning. Machine learning can help researchers identify promising perovskite materials faster and more easily test their viability in devices under different environmental conditions. It also offers the potential to greatly accelerate the development of commercial solar perovskite cells.
Srivastava had never considered using machine learning to study materials for energy applications when she joined UC Davis in 2019, but a conversation with Leite changed her mind.
“I thought it was really cool,” said Srivastava. “I saw a lot of potential in it as a way to get past the ‘Edisonian model’ of experimentation where you hammer at it and keep tweaking little things while running the same experiment over and over.”
Since then, it’s become the focus of her research, which received two best poster awards at the 2020 Materials Research Society (MRS) conference and now an NSF fellowship. Over the three years of GRPF funding, she plans to expand her research to study perovskite alloys with cesium and rubidium, which have been shown to be more stable and durable. With the project, Srivastava hopes to advocate for the field to adopt machine learning.
“I’d love to see people in materials science look at machine learning as not something that computer scientists do, but as another tool in our toolkit to fabricate and characterize materials,” she said. “I’m hoping to help show how to use it so other groups can integrate it into their own projects.”
She also hopes that the data the team collects to train the algorithm can help researchers better understand perovskites in general and help make this next-generation solar technology and commercially-viable reality.
“I would like to eventually move beyond just those [Rb and Cs] compositions and really get a holistic understanding of a lot of different perovskite materials,” she said. “It’s really awesome to be in a field that’s growing at this rate and has so much interest in making something commercially viable that will impact people’s lives.”