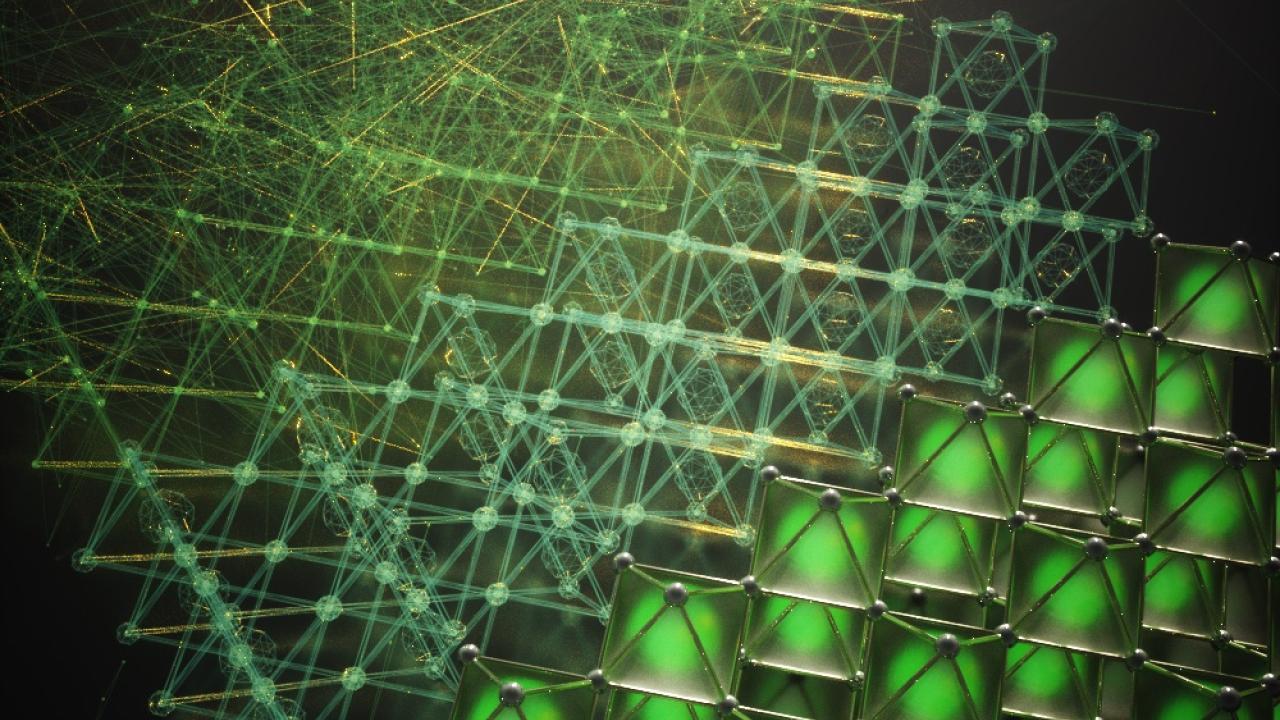
Harnessing AI to Advance Solar Cell Research
New research from Marina Leite, a professor of materials science and engineering at the University of California, Davis, leverages artificial intelligence and machine learning to build foundational knowledge about halide perovskite solar cells.
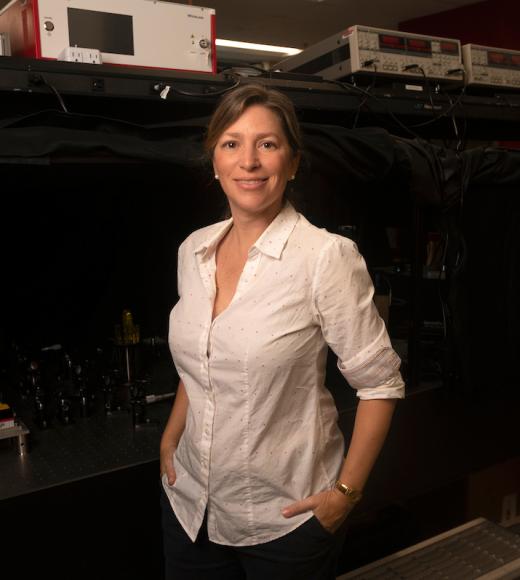
With new funding from the National Science Foundation, Leite and her team, including Ph.D. students Mansha Dubey and Abigail Hering, will combine automated experiments collecting hundreds of thousands of measurements of how humidity and temperature affect perovskite solar cells with a machine-learning-driven analysis.
Leite, who has used artificial intelligence, or AI, in research before, says this will be the first time she has used the tools for this amount of data. Without these technologies, this type of research could take years to complete.
"It could take decades to get to the answer [without AI]," she said. "Instead, we are using tools that can push us in the right direction orders of magnitude faster."
The Perovskite Predicament
The "right direction" in terms of how perovskites interact with carrier transport layer materials, meaning the material in a solar cell that is layered with perovskites to extract the electrical current from the solar energy, during exposure to extreme temperatures and humidities that fluctuate.
The problem, Leite says, is that while perovskites are known to be high-performing and thus promising candidates for solar cells, they are prone to degradation when exposed to environmental changes over time. As a material for solar cells — which will eventually be installed into solar panels that typically sit on rooftops and may experience weather extremes like snow and heat from direct sunlight within a 12-hour period — there is a lot that is still unknown.
One of Dubey's tasks is assembling the high-throughput setup for the physical experiments. The setup involves simultaneously measuring up to 15 unique samples with close humidity and temperature control. A laser excites the charge carriers, and a spectrometer measures the photoluminescence intensity. The experiment is automated and can run for six days at a time, extracting data every fraction of a second.
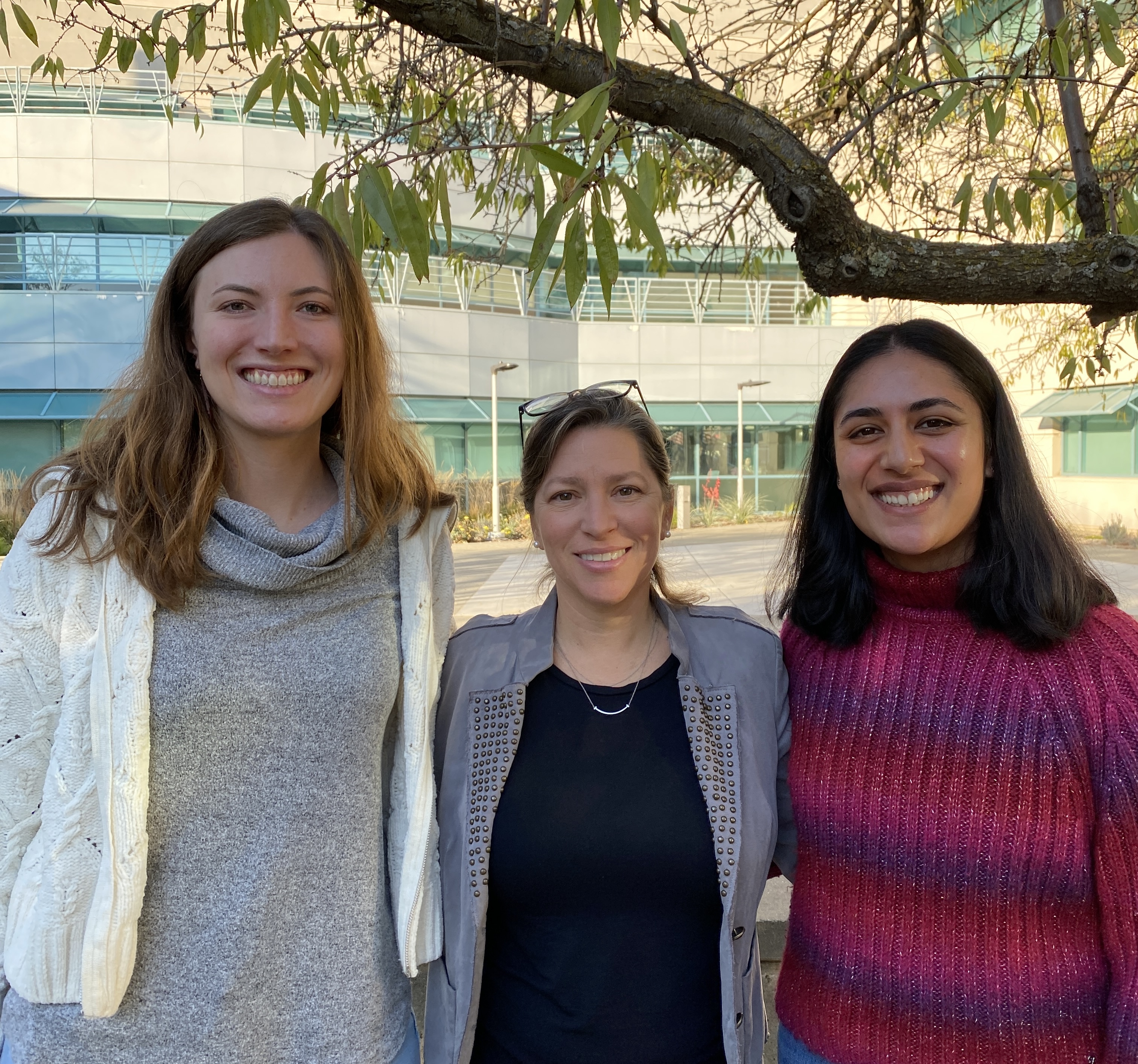
"We have limited knowledge about charge carrier dynamics in perovskites at ambient conditions, so when you add varying temperature and humidity and the interfacial layers to the mix, the complexity of the material increases exponentially," said Dubey. "The goal is to establish the links between all these different variables, deconvolute the effects of temperature and humidity and understand what is going on at an atomic level."
The experiments will show, in real time, whether there are changes to the interface, if those changes are for the better or worse, and if the changes are reversible.
Advancing Materials Science with AI
Once all the data is acquired, it will train a selection of machine learning models, which will be directed to find correlations between the environmental conditions and the different materials. The model will help the team build on the foundational research of perovskites and determine where to go next with the research to create better, more efficient solar cells.
"I truly believe that machine learning will be able to help [to develop next-generation solar cell technology] and advance materials research," said Leite. "We just need to provide it with the right input information."