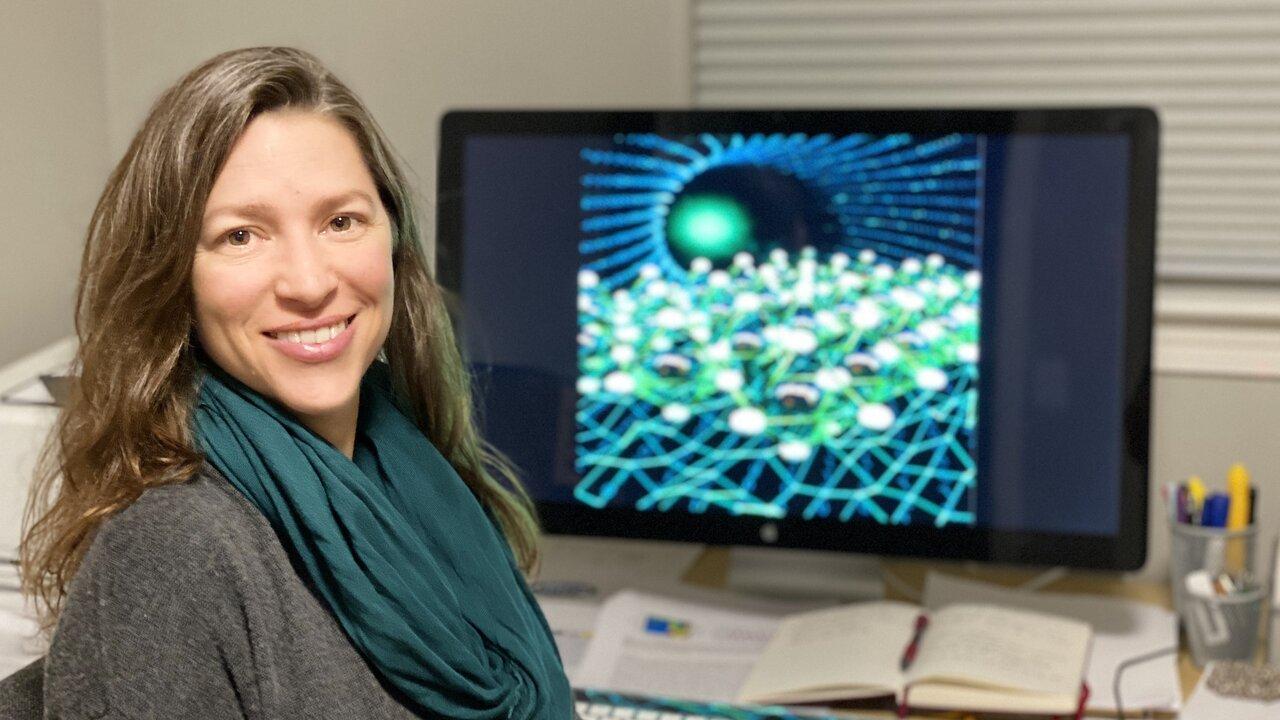
Applying machine learning to renewable energy
Materials science and engineering associate professor Marina Leite thinks machine learning is key to the next big breakthrough in renewable energy. With a new three-year grant from the National Science Foundation, Leite will use machine learning techniques to study perovskite solar cells, a class of highly efficient but volatile devices, to find the optimal conditions to run them reliably.
Perovskites are an organic/inorganic hybrid material used to make solar cells and other optoelectronic devices, which turn light into electricity. To many including Leite, perovskites are the future for renewable energy because they’re extremely efficient and inexpensive to produce, giving them the potential to make solar power accessible and affordable. However, they are highly unstable materials and can quickly degrade under a range of conditions including light, temperature, humidity, oxygen and electrical bias.
In other words, to make perovskite solar cells work reliably for a long period of time, the device needs to operate under a very specific set of circumstances. Because there are so many factors involved that could degrade the material independently or in combination, Leite thinks finding the “goldilocks zone” of ideal conditions is a perfect application for machine learning.
“The number of variables is so enormous that the conventional trial-and-error experimental approach would not give us the answers we are looking for in a timely manner,” she said. “Thus, we need to realize algorithms that will enable us to predict material behavior and device performance by using a suite of high-throughput optical measurement techniques.”
In this project, the team will study metal halide perovskites, which have a unique molecular structure that gives them optimal for solar cells. They will collect data on how the material responds to different conditions and use that data to train a machine learning algorithm, which will help the team determine ideal operating conditions. The team will then conduct real-world tests with perovskite solar cells to validate and refine the algorithm.
Leite thinks the team could establish a framework to quickly diagnose the stability of future perovskite-based devices, as well as a better understanding of the fundamental physical processes that take place within these materials. This knowledge will advance optoelectronic technology and help make renewable energy more accessible to all.
The project, titled “Tackling Instability in Perovskite Solar Cells Through Machine Learning,” began funding September 1.